Implementing AI for Donor Retention: The Complete Playbook
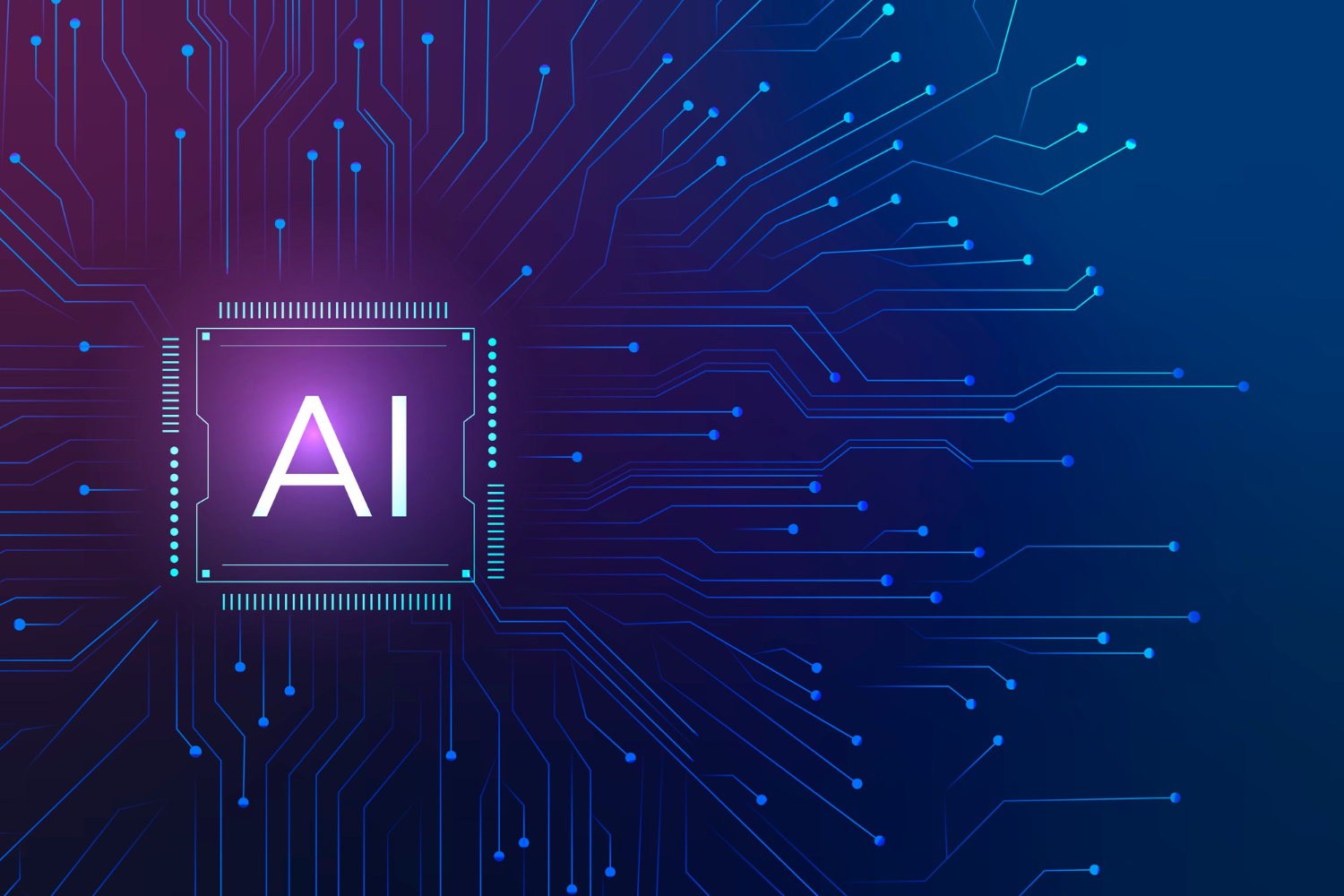
Introduction
The data tells a sobering story: most nonprofits lose 55-70% of their first-time donors before a second gift. With acquisition costs rising, this donor churn represents not just lost revenue but wasted acquisition investment.
Artificial intelligence is changing this equation. Nonprofits implementing AI-powered retention strategies are seeing dramatic improvements, with some organizations doubling their retention rates in less than a year.
This comprehensive playbook will walk you through exactly how to implement AI for donor retention, from initial assessment to full deployment, with practical steps for organizations of any size.
Why Traditional Retention Strategies Fall Short
Before diving into AI solutions, it's important to understand why conventional approaches often fail:
- One-size-fits-all communication doesn't address individual donor motivations
- Reactive retention efforts (reaching out after donors have lapsed) come too late
- Manual segmentation misses subtle patterns that predict donor behavior
- Inconsistent outreach timing means messages often arrive at suboptimal moments
- Limited staff capacity prevents personalized attention to all donor relationships
AI addresses these limitations by analyzing vast amounts of donor data to predict behavior, personalize communications, and optimize timing—at scale, without requiring additional staff.
The AI Retention Framework
Our framework for implementing AI-powered donor retention consists of five core components:
- Predictive Churn Modeling - Identifying donors at risk before they lapse
- Behavioral Segmentation - Creating dynamic groups based on engagement patterns
- Content Personalization - Tailoring communications to individual interests
- Outreach Optimization - Determining the best timing and channels for each donor
- Continuous Learning - Improving the system through ongoing analysis
Let's explore how to implement each component.
Step 1: Predictive Churn Modeling
Predictive churn models use historical donor data to identify patterns that precede a lapsed donation, allowing you to intervene proactively.
Implementation Process:
- Data Preparation:
- Gather 2-3 years of donor history (giving, email engagement, event attendance, etc.)
- Identify which donors have lapsed vs. remained active
- Clean and normalize your data (remove duplicates, standardize formats)
- Select a Predictive Tool:
- Entry level: Use the predictive analytics features in your CRM (many now offer basic AI capabilities)
- Mid-level: Implement a dedicated solution like RetentionIQ or Donor Predict
- Identify Key Indicators:The most predictive signals of donor churn typically include:
- Declining email engagement over time
- Decreased website visits
- Reduced social media interaction
- Changes in giving frequency or amounts
- Missed event attendance compared to previous years
- Create Risk Scoring:Develop a system that rates each donor's churn risk on a scale (e.g., 1-100), with clear thresholds for intervention.
- Test and Refine:
- Run your model against historical data to check accuracy
- Start with a small donor segment to validate predictions
- Refine based on actual retention outcomes
Step 2: Behavioral Segmentation
AI-powered behavioral segmentation goes beyond static categories (like giving level) to group donors based on engagement patterns and preferences.
Implementation Process:
- Identify Engagement Metrics:Track behaviors across channels, including:
- Email opens, clicks, and replies
- Website visits and page views
- Social media engagement
- Event attendance
- Donation patterns (frequency, amounts, restrictions)
- Select a Segmentation Approach:
- Entry level: Use rules-based segmentation enhanced with engagement scores
- Mid-level: Implement a marketing automation platform with behavioral tracking
- Create Dynamic Segments:Common AI-driven segments include:
- Content enthusiasts (high engagement, low giving)
- Passionate supporters (high engagement, regular giving)
- Quiet givers (low engagement, consistent giving)
- At-risk supporters (declining engagement and giving)
- Comeback opportunities (previously lapsed, showing new interest)
- Align Retention Strategies: Develop tailored retention approaches for each behavioral segment.
Step 3: Content Personalization
AI enables true content personalization by matching donors with the information most relevant to their interests and motivations.
Implementation Process:
- Content Categorization:
- Tag all your content (stories, updates, videos) by program area, impact type, and emotional appeal
- Create a content library organized by these tags
- Interest Identification:
- Use AI to analyze which content each donor engages with
- Look for patterns in donation designations and survey responses
- Track website browsing behavior when possible
- Personalization Implementation:
- Entry level: Send different email versions to major interest segments
- Mid-level: Use dynamic content blocks that change based on donor profiles
- Advanced: Create fully personalized communications with AI-selected content for each donor
- Progressive Refinement: As donors engage with content, continuously update their interest profiles to improve future personalization.
Step 4: Outreach Optimization
AI excels at determining when, how, and how often to communicate with each donor for maximum engagement.
Implementation Process:
- Timing Analysis:
- Analyze when each donor typically opens emails, visits your website, or makes donations
- Identify seasonal patterns in individual giving and engagement
- Channel Preference Detection:
- Track which communication channels each donor responds to best
- Note differences in engagement across email, direct mail, SMS, social media, etc.
- Frequency Calibration:
- Test different contact frequencies to find the optimal cadence for each donor segment
- Look for signs of content fatigue vs. engagement boost
- Implementation Options:
- Entry level: Schedule sends based on broad timing patterns (day of week, time of day)
- Mid-level: Use marketing automation with send-time optimization
- Advanced: Implement AI tools that determine custom timing for each communication
Step 5: Continuous Learning
The most effective AI retention systems continuously improve based on new data and outcomes.
Implementation Process:
- Retention Metrics Definition: Establish clear metrics to track, including:
- Retention rates by segment and acquisition source
- Time to second gift
- Lifetime value progression
- Engagement score trends
- A/B Testing Framework:
- Test different interventions for at-risk donors
- Compare retention rates across approaches
- Document what works for different segments
- Feedback Loop Creation:
- Feed results back into your predictive models
- Update segmentation based on new behavior patterns
- Refine content personalization based on engagement data
- Ongoing Optimization:
- Establish a regular review cadence (monthly is ideal)
- Document insights and evolve your approach
- Continuously test new retention hypotheses
Conclusion
AI-powered donor retention represents one of the highest-ROI investments a nonprofit can make in today's competitive fundraising environment. By implementing even basic predictive models and personalization, organizations can significantly reduce donor churn while building stronger, more meaningful supporter relationships.
The key is to start small, focus on quick wins, and continuously refine your approach based on results. With each improvement, your retention system will become more effective at keeping donors engaged for the long term.
Ready to implement AI for donor retention at your organization?
Contact KNDR for a free consultation to discuss your specific needs and goals.
